The Shifting Boundaries of Point Cloud Processing Time
与处理点云相关的时间问题非常令人沮丧,但它们并不是什么新鲜事物。根据Huibert-Jan Lekkerkerk的说法,不断变化的界限意味着我们注定要永远玩等待游戏188金宝搏特邀, in his latest column.
尽管被贸易水文测量员,我understand geospatial surveyors’ issues with point clouds all too well. Hydrography, as you may know, largely revolves around measuring depths, i.e. ‘bathymetry’. Ever since joining that industry in the 1990s I have been involved in what hydrographic surveyors call multibeam bathymetry: the underwater variant of Lidar altimetry. The first instrument I used was a Reson 9001 capable of giving 60 depths per measurement (‘swathe’) at around 15 swathes per second. In other words, it produced around 900 depths per second. Today such systems achieve up to 1,000 depths per swathe and 60 swathes per second, totalling 60,000 depths per second. But back then, even 900 depths per second posed a big problem as far as processing was concerned. A full day of surveys would take around half a day to process using our Pentium 75 processors with 8MB of memory (which back then were state-of-the-art!). Data transfer was done using 4-speed CD-ROMs or portable hard disks of 500Mb.
Although the data was gathered as a point cloud, we could not process it that way so it was gridded using a 1x1m2bin grid for example – and even that would tax the computer at times. Around the turn of the millennium, I got into a discussion with a software vendor who told me his software could handle big datasets without a problem. I replied that our project entailed conducting 20-minute surveys using a system that provided 256 depths per swathe at 40 swathes per second. In other words, a small survey would produce over 12 million points that needed to be visualized. Strangely enough, I never heard from that vendor again…!
Today, multibeam echosounding, Lidar or photogrammetry make those numbers seem laughable. We now have the advantage that computers have become much faster, combined with cheaper data storage on a much larger scale… and yet the problems are still the same. For example, just a few weeks ago I asked a student to prepare a presentation on photogrammetry. Since many of the students at our hydrographic college had returned from their traineeship explaining how they augmented their hydrographic surveys with drone data to connect the land and water parts, we had just purchased our first A1-class drone with a meagre 12MP camera and basic GPS/Glonass/Galileo on board. This particular student had done many such surveys during his recent traineeship on a harbour extension project and had even acquired his drone pilot licence. So to demonstrate the processing workflow, he conducted a survey of a quayside close to the college. He took around 140 photographs at 12MP, created some ground control points which he surveyed using the college’s RTK system and started to process his small (max. 30-minute) survey. He ended up with a really impressive 3D model of around 12 million points, but only after a considerable wait (albeit less than half a day). The main difference between now and two decades ago is that he didn’t need a state-of-the-art computer, but instead used his own trusty gaming laptop.
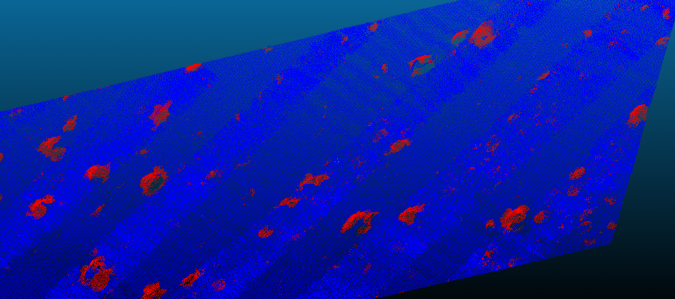
I had arranged for one of my bachelor students to give a guest lecture to the same class. For his current thesis work on the use of artificial intelligence (AI) for detecting boulders in multibeam echosounder point clouds, he has built some software in Python and is now assessing the accuracy of his tool. He had obtained a dataset of a few million datapoints and split that up into a training set and a ‘regular’ set. In the guest lecture, he asked the students to help him verify the accuracy of his software by manually ‘clicking’ on what they thought were boulders using the hydrographic QPS Qimera cloud processing software. On their regular gaming laptops, it took them around 15 minutes for the entire dataset. He then ran his (non-optimized) software on 10% of the dataset, because that was the most his computer could handle at once. The run took around five minutes. Besides discovering that he had to fill a five-minute void of lecture time while watching the status bar slowly advance, he demonstrated that performing elaborate processing on a point cloud takes time, even today.
In conclusion, the time issues associated with processing point clouds have been around for decades, and whenever we think we are about to catch up, the boundaries shift again: new sensors, new requirements or new tools. The only solution is to keep looking for the balance between ‘acceptable’ processing time and customer satisfaction. When it comes to processing point cloud data, it seems we’re destined to forever play the waiting game…
使您的收件箱更有趣。Add some geo.
Receive a weekly summary of the biggest news, along with the best stories, case studies, and key market insights.
免费注册