Lidar or Photogrammetry? Why Not Use Both?
How Data Fusion Can Improve Point Cloud Quality
Is it possible to enhance point cloud accuracy and density by merging Lidar with photogrammetry technologies? Lidar data can penetrate trees and measure shadow areas to produce a very accurate point cloud. Passive imaging cameras derive a more detailed 3D model and encode point clouds with multispectral information, resulting in a useful coloured point cloud classification. What if were possible to merge these technologies? What effect might neutral density filters have on point cloud colours? This article explores quantitative and qualitative point cloud enhancement in more detail.
无人驾驶飞行器(无人机或“无人机”)become increasingly popular for many environmental applications, delivering point cloud digital surface models (DSMs) and orthoimagery. The accuracy of datasets generated from drone flights depends on the data capturing methods, from active Lidar sensors or from passive remote-sensing sensors (cameras). Point cloud datasets usually contain a considerable number of undesirable irregularities, such as strong variability of local point density, missing data, overlapping points and noise. This recent study by the GeoSpatial Laboratory of the Faculty of Letters and Human Sciences at the Lebanese University applied various methods for point cloud quality enhancement and also conducted field experiments to reduce the undesirable irregularities of point clouds.
Novel point cloud quality improvement method
High-resolution and high-density point clouds play an important role in many applications such as deformation monitoring studies and indoor and outdoor mapping. Several studies have compared Lidar and structure from motion (SfM) photogrammetry techniques and found that Lidar datasets are more accurate because SfM-derived results are variable. One advantage of SfM photogrammetry is the encoding of point clouds with multispectral information, which is a useful coloured point cloud classification. However, Lidar data can penetrate trees and measure shadow areas to produce a very accurate point cloud. Lidar scanners provide a relatively accurate 3D point cloud, in contrast to passive imaging cameras that derive a more detailed 3D model of the real world.
Geospatial technology users often question what to choose for scanning an urban area: Lidar or photogrammetry? But what happens when both technologies are utilized? Landscape and street photographers use neutral density (ND) filters to enhance their images, so why not apply this method to photogrammetry?
地理空间实验室教师的信s and Human Sciences at the Lebanese University has developed a framework to introduce a novel point cloud quality improvement method based on Lidar and SfM fusion and the integration of neutral density filters on passive sensors. Two improvements are implemented: 1) Increase in Lidar point cloud density and SfM point cloud accuracy, and 2) Enhancement of point cloud colours. Quantitative and qualitative point cloud enhancement has been explored by testing point cloud fusion and the effect of four neutral density filters (ND-4, ND-8, ND-16 and ND-32) on point cloud colouring.
Fusion
点云用户从许多学科都有显示an interest in fusing Lidar and photogrammetric data to qualitatively enhance point clouds. The fusion of both SfM and Lidar data then increases the usability and benefits of the datasets. In this study, the researchers produced a point cloud exploiting Lidar and SfM techniques and utilized the Iterative Closest Point (ICP) method to fuse Lidar and photogrammetry point clouds into one mapping frame.
Since drone images are affected by the sun’s rays, photogrammetrists advise that missions are conducted at lower sun intensity. To reduce the quantity of sunlight, professional photographers use ND filters. Due to a lack of literature and projects referencing the use of ND filters in photogrammetry, this study is among the first to test the effect of such filters on drone dataset enhancement.
Point cloud accuracy and density enhancement
航空激光雷达数据集和的运用photogrammetry could be done by GPS-RTK or GPS-PPK, in the same coordinate systems. The iterative closest point (ICP) algorithm helped in reducing the distance between the source Lidar point clouds and the photogrammetry closest point pairs, allowing for greater accuracy.
The main advantage of the ICP method is the direct use of measurement data, as there is no need for interpolation or other data modification. It attempts to find the optimal transformation between two point clouds by minimizing the average distance of corresponding point pairs.
Following an ICP algorithm, both Lidar and SfM-based point clouds were merged into one fusion point cloud file (Figure 1).
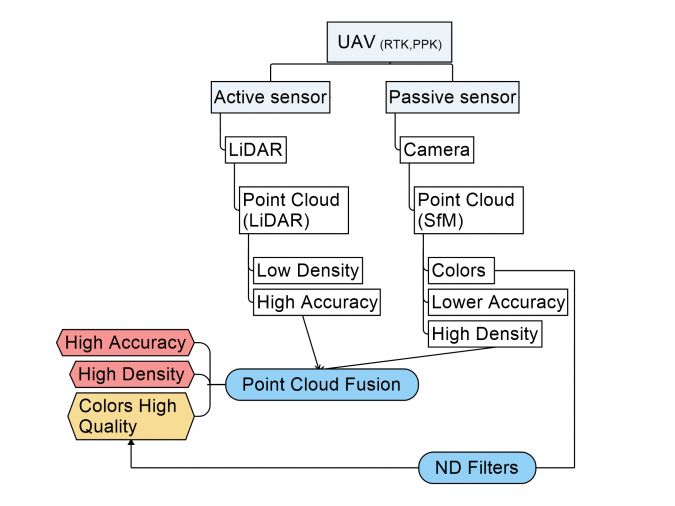
The new merged point cloud is characterized by the high accuracy of Lidar and by the high density of SfM point clouds. In an urban area at a flight height of 150m, the researchers scanned 845,669 Lidar points. On the same aerial platform, a camera captured 343 frames. Applying SfM technology generated a point cloud seven times greater than Lidar alone, with a total of six million points (Figure 2).
A visual qualitative analysis of Figure 2 uses criteria such as shape, form and density, while the quantitative approach uses point cloud density. Point cloud visualization of different datasets shows definite differences in terms of point density, details and noise. Using photogrammetry and a Lidar point cloud to detect building boundaries has limitations due to the noise and various uncertainties of photogrammetric point clouds. Moreover, as the Lidar technology is an active remote sensing technology based on laser beams, it detects thin structures such as electric wires, fences, etc.
The visual analysis of linear structures of the building’s boundaries has a very accurate geometric form in Lidar. In contrast, in SfM, buildings and high trees are affected by the shadows and have no point clouds areas. The difference between Lidar and SfM is due to shadows caused by buildings and trees and to trees being omitted when they have no leaves (Figure 2). The ICP algorithm solves this problem by filling these empty areas from the Lidar point cloud to be interpolated in the merged one.
In SfM, shrubs, small trees, embankments and solar panels on roofs were detected due to the high ground sampling distance (GSD) of 3cm. The merged datasets also have the availability to visualize these urban structures. SfM methods are not capable of penetrating vegetation cover.
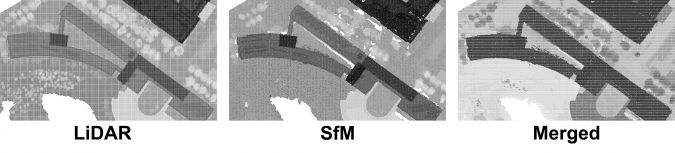
The generated fusion point clouds enhanced the quality of the SfM point cloud by taking the accuracy from Lidar datasets, and enhanced the quality of the Lidar point cloud by increasing its density from the SfM point cloud (Doumit, 2020).
Point cloud colour enhancement
Neutral density filters earn their name from the fact that they do not affect the colour of light passing through them. Using ND filters can decrease light by one, two, three or more stops, allowing a slow shutter speed (Bryan 2004).
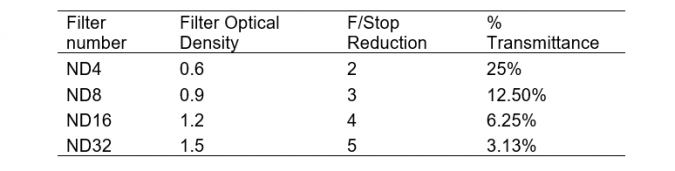
Table 1 shows that the filter's optical density and the stop reduction increase with the filter number, and have a high influence on transmittance. To test the ND filters, five flights were conducted of the same area using: no filter, ND-4, ND-8, ND-16 and ND-32. Processing resulted in the output of five point clouds with the same specifications but with different colours, radiometry and textures. The no-filter point cloud acts as a basis for comparison between the filtered point clouds. In a visual interpretation (Figure 3), high texture details were detected in point clouds generated using ND-4, ND-8 and ND-16, whereas in the point cloud without a filter and with ND-32, the details are less clear.
Brick roofs, tree leaves, fences and other details are highlighted in ND-8 and ND-16 and obscured in all other point clouds. The variations in colour brightness and contrast enable the identification of the target with other elements like shape, size, etc. The texture is the arrangement and frequency of tonal variations from rough to smooth; smooth textures have uniform tonal variations like the point cloud without a filter and ND-32, whereas rough textures have abruptly tonal variations as visible in the results using ND-4, ND-8 and ND-16 (Doumit & Abou Chakra, 2020).
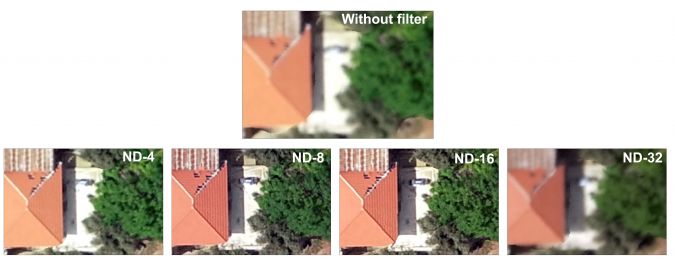
In the visual image interpretation, ND-8 and ND-16 were selected as the better-coloured point clouds. If the results shown in Figure 3 were to be ranked from most detailed to least detailed, the classification would be as follows: ND-8, ND-16, ND-4 and ND-32. The filter ND-32 should be used only if high sun intensity occurs during photogrammetry missions.
Conclusion
The researchers tested UAV-generated Lidar and photogrammetry point clouds to assess the enhancement of point cloud quality. ICP was utilized for merging the two point clouds into one mapping frame in order to enhance photogrammetry point cloud accuracy and to add more density to the Lidar point cloud.
Producing a Lidar, an SfM photogrammetry and a merged point cloud allowed for comparison in terms of quality. The results show that gaps in the photogrammetry point clouds and the low density of Lidar point clouds could be enhanced after the data fusion process. This study also considered the effect of neutral density filters for point cloud colour enhancement.
In conclusion, for better mapping of large-scale urban areas, it is preferable to use a fusion of Lidar and photogrammetry point cloud data because Lidar can penetrate dense vegetation and produce ground points accurately, while image-based matching point clouds can give dense high texture and high spatial resolution datasets. Given this, it is the author’s hope that new software will soon become available for merging photogrammetry-generated point clouds with Lidar point clouds into one final enhanced dataset.
Further reading
- Bryan, F.P. (2004). Understanding exposure: how to shoot great photographs with a film or digital camera. Amphoto Books. ISBN 978-08174-6300-7.
- Doumit, J. A. (2020). Lidar and SfM point cloud fusion effects in the generation of urban digital surface models.BRICS Journal of Educational Research, Vol.10, pp. 40-44.https://www.bricsjer.com/index.php/brics/article/view/232.
- Doumit, J.A, & Abou Chakra, C. A. (2020). The effect of Neutral Density Filters on drones orthomosaics classifications for land-use mapping. OSF Preprints.https://doi.org/10.31219/osf.io/dw6ju
Make your inbox more interesting.Add some geo.
Keep abreast of news, developments and technological advancement in the geomatics industry.
Sign up for free